New to Honeycomb? Get your free account today.
Seems like you can’t throw a rock without hitting an announcement about a Model Context Protocol server release from your favorite application or developer tool. While I could just write a couple hundred words about the Honeycomb MCP server, I’d rather walk you through the experience of building it, some of the challenges and successes we’ve seen while building and using it, and talk through what’s next. It should be pretty exciting, so strap in!
(I get it, skip the explanation and show me the good stuff)
Help! I studiously avoid everything involving AI…
…and/or I just got out of a time portal from 2021. What’s Model Context Protocol?
An exhaustive description of what MCP is is slightly out of scope for this blog post, but I do want to give you a brief overview. First, recall how modern AI tools work—specifically, Large Language Models. In an extremely broad sense, LLMs are designed to predict ‘what token should come next’ in response to input. If you’ve ever played around with markov chain generators, this concept shouldn’t be too unapproachable. Where LLMs shine, though, is that they’re more or less able to function as non-deterministic, natural language computers. Through prompting—the inputs you provide to a LLM—you’re able to control and specify the output of the model, even going so far as to coerce it to output in a specific format.
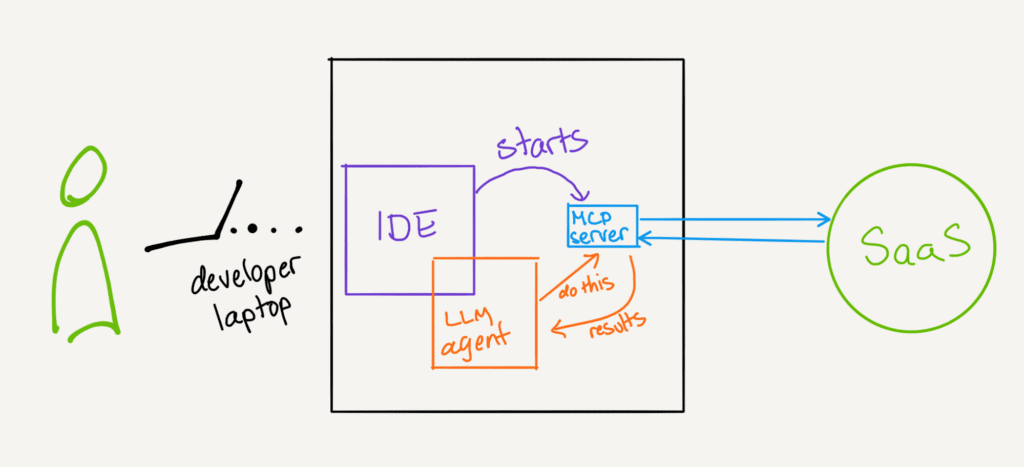
This notion led to the development of tool calling in LLMs. The idea of tool calls is that, through crafted prompts, an LLM can both read and write to JSON objects that obey a defined schema, provided in their context. The context is, effectively, the ‘short term memory’ of an LLM. Tool calling opened up a lot of doors for generative AI, because it gave developers a way to interface with the model. Developers could write tools that could expose functions through JSON Schema, LLM interface authors could provide those tool definitions to the model context in a conventional way, and the LLM could kinda figure the rest out on the fly.
A simple example of this is a calculator. LLMs aren’t great at doing math, but if you provide a ‘calculator’ tool that exposes a schema of operations—add, subtract, etc.—and write some code to perform the calculation, then the LLM can determine what to do when a user asks “What’s 9394792 + 190100158?” It calls the calculator tool with the appropriate parameters, gets the result, and displays it to the user. These tool calls can be then chained by the LLM in order to perform more complex operations.
Wait, that sounds extremely stupid.
I mean, kinda, sure.
Tool calling is great because it allows you to build systems, which is the point of this job, I think. LLMs aren’t great at doing things that aren’t… well, token prediction or classification or other machine learning stuff. It doesn’t make sense to try to ‘teach’ an LLM to be ‘good at calculations.’ We don’t need the ML model to do that if we can give it a calculator, right?
In addition, while passing JSON around seems pretty inelegant, it’s one of those things that LLMs have a great intrinsic sense for because there’s so much JSON in the training data. Models are ridiculously good at parsing and writing valid JSON, partially because it’s simple, and partially because it’s ubiquitous. Why not use something that already exists and everyone understands pretty well for the task of “having a LLM talk to things that aren’t the LLM and also aren’t the user?”
In late 2024, Anthropic introduced the Model Context Protocol, a specification for writing servers and clients that respected this tool calling approach. MCP has become an overnight hit (so big in fact that there are already competing standards, wee!) because it solves a pretty big pain point for developers who would like to connect AI to other systems—which, it turns out, is a lot of developers.
Broadly speaking, two types of MCP servers have proliferated since the announcement. The first are tools that connect the LLM with ‘local’ functionality on your computer. This is stuff like making web searches, taking screenshots, accessing the file system, and so forth. The second are tools that connect the LLM with ‘remote’ functionality—think talking to GitHub, remote databases, or other SaaS applications. It’s genuinely quite handy; you can set up a GitHub MCP server, give it an access token, and ask it to summarize all of your pending PRs, issues, or so forth.
So, wait, this is just a way to wrap existing APIs?
More or less. It’s certainly a lot easier to write and run MCP servers and plug them into various chat interfaces than it is to actually write the code to stitch this stuff together yourself.
Enter: Honeycomb MCP.
I had some free time at the end of last year and knocked out a Honeycomb MCP server. It was fairly basic: it exposed our query interface, so you could ask Claude things like, “What’s the slowest route in my production environment?” and it would figure out how to write that query, issue it through our Query Data API, then retrieve the results and pick that info out. It worked well enough that we decided to spend a week really working on it and brought it into our GitHub organization. It’s, at best, a preview—I’ll discuss more why later—but if you’re a Honeycomb Enterprise customer, you can download and use it today.
What can you do with Honeycomb MCP? Quite a bit, really. We’ve built tools that allow models to access all of the resources in your environments—boards, triggers, SLOs, queries, and so forth. When running the MCP server in an appropriate client, like Claude Desktop, VS Code, or Cursor, you can give agents open-ended tasks and it can use these tools to accomplish them.
For example, if you have an SLO that’s burning down, Cursor agent can inspect that SLO and perform investigations in Honeycomb to find more data that it can use in conjunction with analysis of your codebase to find and fix bugs or improve performance. A really neat trick is asking the agent to improve the instrumentation of a new or existing service based on what is seen in other services (or a specific one)—it can use Honeycomb to figure out specific idioms and attributes that are already in use, then follow those patterns when editing more code. It’s very good at using Honeycomb in conjunction with other data added to an agent context—for instance, OpenTelemetry Semantic Conventions—to discover opportunities for telemetry refactoring (e.g., converting existing log-based telemetry to spans).
Sounds great! What’s the catch?
In a lot of blogs, this would be the part where we wrapped up and sent you on your way. Instead, I’m going to go a little more in-depth on the rough edges we discovered while building Honeycomb MCP.
Most APIs aren’t designed for LLMs, including ours.
Easily the biggest challenge we’ve faced with the MCP server is the sheer amount of tokens that our query data API returns for anything more than extremely basic queries. While this can be somewhat worked around by prompting, it’s really only the tip of the iceberg. Some Honeycomb accounts can have tens of thousands of columns, thousands of triggers, and hundreds of datasets. It’s extremely easy for an agent to get itself in a doom loop of queries and hallucinations where it constantly forgets the name of attributes, gets confused about dataset names, and more.
I expect that this is going to be a huge problem for other SaaS tools to tackle as they build MCP servers and other integrations; LLM interface design is unique! The kind of response that you return through your standard JSON API that’s suitable for programmatic access is probably not what you should return to an LLM. MCP servers do provide a nice abstraction layer for you to address this in flight—you can edit the responses before you return them to simplify data structures, remove unneeded fields, and so forth.
All of your problems are idiosyncratic problems if you’re not a coward
Honeycomb’s query structures aren’t basic SQL, and in fact are a bit of an odd duck. There is significantly more training data about “how to query” than there is “how to query Honeycomb,” and we don’t necessarily have a great way to parse and validate queries other than ‘sending them to Honeycomb and hoping it works.’ While we do provide feedback to the model when this happens, it’s still frustrating to have the agent cycle through multiple subtly tweaked Honeycomb queries only to give up and drastically simplify the query or try ‘something else’ that it thinks will work better.
This actually gets to a much more pernicious problem that I’ve personally seen with coding-oriented assistants—the goal-seeking behavior of the model training optimizes for “doing something” rather than “doing the right thing.” You can replicate this yourself fairly easily—try to run something that requires an external API token to work, but don’t provide the token. You’d perhaps expect that the model would stop and tell you ‘hey, put the token in!’ but they’ll often simply start changing the code to mock the token, then start writing mock responses. The goals are too tightly oriented around ‘get the software to build/run’ rather than holistically addressing issues.
This smacks headlong into our query specification issue. The model will do something subtly wrong, get an error, make a small tweak, get another error, then pretty much give up and drastically simplify the query. You need to do some hand-holding to make sure it doesn’t go off on a flight of fancy. The more precise your prompts, the better it does. One trick: use memory files to make sure the model has access to known data (such as important board, environment, and attribute names) so that it doesn’t have to guess or constantly go fetch columns. “Wait, why not send the query spec in the tool description?” Great plan, but this has the side effect of making the ‘responses are too chatty’ problem even worse, and blows out the context window, leading to even more hallucinations.
Things you learn when you build an API on an API
All unhappy families are different in their own way, and our API is no different. There are some features it doesn’t support at all, there’s some confusing cruft in there, and we’re in the process of improving it in general—but it’s a work in progress. This leads to unfortunate realizations like, “Wait, API keys are scoped to an environment and not a user, huh…” which forced us to come up with some creative solutions allowing you to use the server with multiple environments.
We also found a lot of things that maybe aren’t idiosyncratic, but are interesting. For example, if you want the LLM to analyze a query, it can be a lot more efficient (in terms of tokens) to have the model follow the query image link in the response, ‘look’ at it using vision, and interpret that rather than trying to parse the JSON.
This all might come off as a bit negative. Am I saying that the Honeycomb MCP server sucks? No, not at all. I think it works remarkably well, especially for the amount of work that’s gone into it, and we’d love for people to try it out. What I really want to talk about is what we’re gonna do next.
Honeycomb MCP: the future
I believe that AI assistants for coding are here to stay, and I’m excited about the work that’s happening around connecting tools to LLMs. However, I think the current paradigm kinda sucks. It’s silly to have everyone download and run a Python script or TypeScript package just to talk to an existing public API. The MCP spec comes through for us here with its SSE (Server Sent Events) and HTTP streaming transports. This allows services to build, run, and host MCP servers remotely. No juggling API keys or dealing with weird path issues, just plug in a URL and away you go.
We plan to begin experimenting with a hosted Honeycomb MCP server that we can offer to more than just our Enterprise clients. Ideally, you’ll go into your account page and get a unique URL that you can plug in to a MCP client and gain access to all of your teams, environments, boards, triggers, and more.
As a part of this, we can optimize responses and tool schemas to work best with LLMs. We should be able to tailor the output from Honeycomb to language models, compacting large responses into more efficient ones, and relying on multimodality to encode dense information into images (either through image links or base64—we’ll have to see what works best) or other more compact representations.
Does this sound like something you’d be interested in? We’d love to hear from you as we build out this feature. In fact, you can sign up here to be the first to learn about early access opportunities. We’d especially love to hear from you if you’re already using MCP servers from other tools or platforms, and how you’re interfacing with them. Please be on the lookout for more information in the coming months—and if you’re a Honeycomb Enterprise customer that wants to try it out today, head on over to GitHub and try it out.